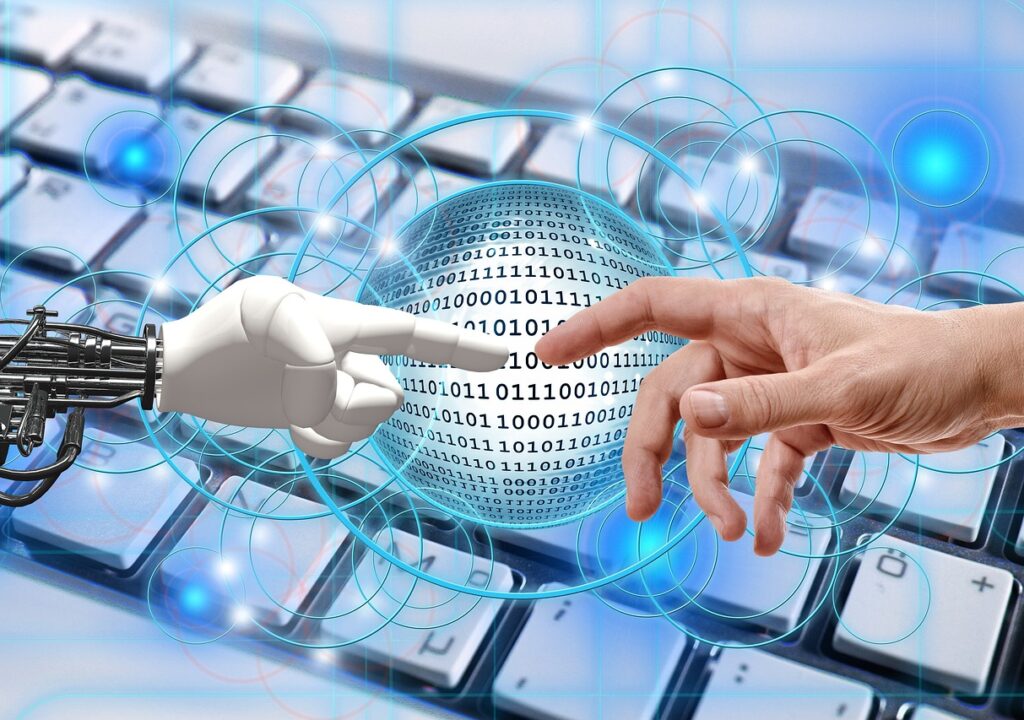
The interplay between co-intelligence and artificial intelligence (AI) has sparked significant interest in recent years, creating a dynamic intersection of human collaboration and advanced computational capabilities. Co-intelligence, a concept rooted in collective human efforts and the ability to work together effectively, when combined with the technological prowess of AI, offers a transformative potential set to revolutionise various domains, inspiring a profound sense of hope and a wide range of possibilities.
Author: Johanna Heinonen
Understanding Co-Intelligence and AI
Co-intelligence enhances collective intelligence through collaboration, inclusivity, and diverse perspectives. It focuses on people working together, understanding and respecting each other’s ideas to solve problems and make decisions, emphasising the quality of relationships and interactions (Krimerman 2004). AI technologies like machine learning, natural language processing, robotics, and computer vision revolutionise industries by automating tasks and analysing large data sets. Mollick (2024) defines AI as simulating human intelligence processes like learning and reasoning.
Combining co-intelligence with AI merges human creativity and social skills with computational power, leading to transformative outcomes. This synergy enhances decision-making by quickly processing vast data and adding diverse perspectives, making decisions more robust. It also improves problem-solving by integrating multidisciplinary expertise with AI-driven insights (Page 2007). Innovation and creativity thrive as co-intelligence fosters idea exchange and AI generates novel ideas (Shneiderman 2022). AI enhances scalability by facilitating global communication and coordination, enabling large-scale collaborative projects and overcoming geographical barriers (Papadopoulos et al. 2021).
Challenges and Considerations
Addressing environmental challenges requires the collective effort of scientists, policymakers, and communities. Co-intelligence can facilitate this collaboration, while AI can analyse environmental data, predict trends, and optimise resource management for more effective and sustainable solutions. By understanding these practical applications, the audience can feel informed and knowledgeable about the potential of co-intelligence and AI integration. (Rolnick ym. 2023.)
Despite the promising potential of integrating co-intelligence and AI, Mollick (2024) emphasises several challenges and considerations. Ethical concerns are paramount, as AI raises questions about privacy, bias, and accountability. For instance, using AI in decision-making processes can lead to biased outcomes if the training data does not represent the entire population. Privacy concerns arise from the collection and use of personal data by AI systems. Ensuring that AI systems are designed and used ethically is not just important. It is a responsibility. This includes addressing fairness, transparency, and accountability issues in AI systems (Floridi et al. 2018).
Co-intelligence can play a role by involving diverse stakeholders in developing and overseeing AI technologies. Accessibility is another critical issue. To fully realise the benefits of co-intelligence and AI, it is essential to ensure that these technologies are accessible to all. This includes addressing digital divides and providing training and resources to underserved communities. Effective integration requires collaboration across disciplines, which can be challenging due to differences in terminology, methodologies, and priorities. Creating frameworks and platforms that facilitate interdisciplinary collaboration is essential. (Wagner et al. 2017.)
Moreover, the relationship between humans and AI must be carefully managed to ensure that AI augments rather than replaces human capabilities. This involves designing AI systems that enhance human creativity, decision-making, and problem-solving. By stressing the need for ethical considerations, the audience can feel responsible and mindful about the use of AI, understanding the importance of its ethical application. (Mollick 2024.)
Strategies and case examples
Fundamental approaches for effective strategies in integrating co-intelligence and AI include designing digital platforms for seamless collaboration, equipping individuals with necessary AI skills through educational programs, and fostering interdisciplinary research. The need for such collaboration is crucial, as it can unite diverse perspectives and priorities, making the audience feel cooperative and united in pursuing AI integration. (Mollick 2024.)
Several case studies illustrate the practical benefits of co-intelligence and AI integration: e.g. IBM’s Project Debater improves debate quality by processing vast amounts of information. The Human Genome Project demonstrates how global collaboration and AI can accelerate genetic discoveries. Intelligent cities optimise services and resource management using AI, co-intelligence, and AI-powered platforms to enhance disaster response through real-time data analysis and coordination. (Brynjolfsson & McAfee 2016.)
Co-intelligence fosters collaborative learning environments where students and educators share knowledge and insights. AI personalises learning experiences, tracks progress, and recommends resources, creating a more engaging and practical educational experience. This integration enhances the quality of education by combining diverse human perspectives with AI-driven analytics and personalised support, making the audience feel engaged and part of the learning process. (Salah El Din & Al Harrasi 2024.) This is also the future of teaching at LAB, making teachers re-evaluate their and student’s roles in virtual working environments.
Future research
Future research in co-intelligence and AI integration offers numerous opportunities. Key areas include optimising human-AI interaction, exploring collaboration frameworks, and applying AI in social and behavioural sciences to address complex social issues (Shneiderman 2022). Addressing ethical concerns and biases in AI systems is crucial, as well as focusing on fairness, transparency, and accountability to build trust (Floridi et al. 2018). Ensuring accessibility involves developing affordable AI tools and addressing digital divides. Additionally, AI and co-intelligence can address environmental challenges through sustainable resource management, climate change mitigation, and biodiversity conservation (Rolnick et al. 2023).
References
Brynjolfsson, E. & McAfee, A. 2016. The second machine age: work, progress, and prosperity in a time of brilliant technologies. W. W. Norton & Company.
Salah El Din, M. & Al Harrasi, N. H. 2024. AI-Driven Transformation in Higher Education: New Frontiers in Administration and Management. In Al Harrasi, N. H. & Salah El Din, M. (Eds.). Utilizing AI for Assessment, Grading, and Feedback in Higher Education. IGI Global. 135-161. Cited 1 July 2024. Availdable at https://doi.org/10.4018/979-8-3693-2145-4.ch006
Floridi, L., Cowls, J., Beltrametti, M., Chatila, R., Chazerand, P., Dignum, V., Luetge, C., Madelin, R., Pagallo, U., Rossi, F., Schafer, B., Valcke, P., & Vayena, E. 2018. AI4People—An Ethical Framework for a Good AI Society: Opportunities, Risks, Principles, and Recommendations. Minds and Machines. Vol. 28(4), 689–707. Cited 1 July 2024. Available at https://doi.org/10.1007/s11023-018-9482-5
Krimerman, L. 2004. The Tao of Democracy: Using Co-Intelligence to Create a World That Works for All [Review of The Tao of Democracy: Using Co-Intelligence to Create a World That Works for All]. Humanity and Society. Vol. 28(3), 352–358.
Mollick, E. 2024. Co-Intelligence: Living and Working with AI. New York: Portfolio/Penguin.
Page, S. E. 2007. The difference : how the power of diversity creates better groups, firms, schools, and societies. Princeton: Princeton University Press.
Papadopoulos, G. T., Antona, M., & Stephanidis, C. 2021. Towards Open and Expandable Cognitive AI Architectures for Large-Scale Multi-Agent Human-Robot Collaborative Learning. IEEE Access. Vol. 9, 73890–73909. Cited 1 July 2024. Available at https://doi.org/10.1109/ACCESS.2021.3080517
Shneiderman, B. 2022. Human-Centered AI. Oxford: Oxford Academic. Cited 1 July 2024. Available at https://doi.org/10.1093/oso/9780192845290.001.0001
Rolnick, D., Donti, P. L., Kaack, L. H., Kochanski, K., Lacoste, A., Sankaran, K., Ross, A. S., Milojevic-Dupont, N., Jaques, N., Waldman-Brown, A., Luccioni, A. S., Maharaj, T., Sherwin, E. D., Mukkavilli, S. K., Kording, K. P., Gomes, C. P., Ng, A. Y., Hassabis, D., Platt, J. C., … Bengio, Y. 2023. Tackling Climate Change with Machine Learning. ACM Computing Surveys. Vol. 55(2), 1–96. Cited 1 July 2024. Available at https://doi.org/10.1145/3485128
Wagner, C., Singer, P., Karimi, F., Pfeffer, J. & Strohmaier, M. 2017. Sampling from Social Networks with Attributes. WWW ’17: Proceedings of the 26th International Conference on World Wide Web. 1181–1190. Cited 1 July 2024. Available at https://doi.org/10.1145/3038912.3052665
Author
Johanna Heinonen is a senior lecturer and RDI specialist at LAB University of Applied Sciences. In particular, the diverse development of tourism, digital communication and customer experience are close to her heart. Also, student experiences and applications of new technologies in teaching inspire her.
Illustration: https://pixabay.com/fi/photos/k%C3%A4si-robotti-ihmisen-n%C3%A4pp%C3%A4imist%C3%B6-3685829/ (Gerd Altmann, Pixabay License)
Reference to this article
Heinonen, J. 2024. Exploring Co-Intelligence and Artificial Intelligence. LAB Pro. Cited and the date of citation. Available at https://www.labopen.fi/lab-pro/exploring-co-intelligence-and-artificial-intelligence/